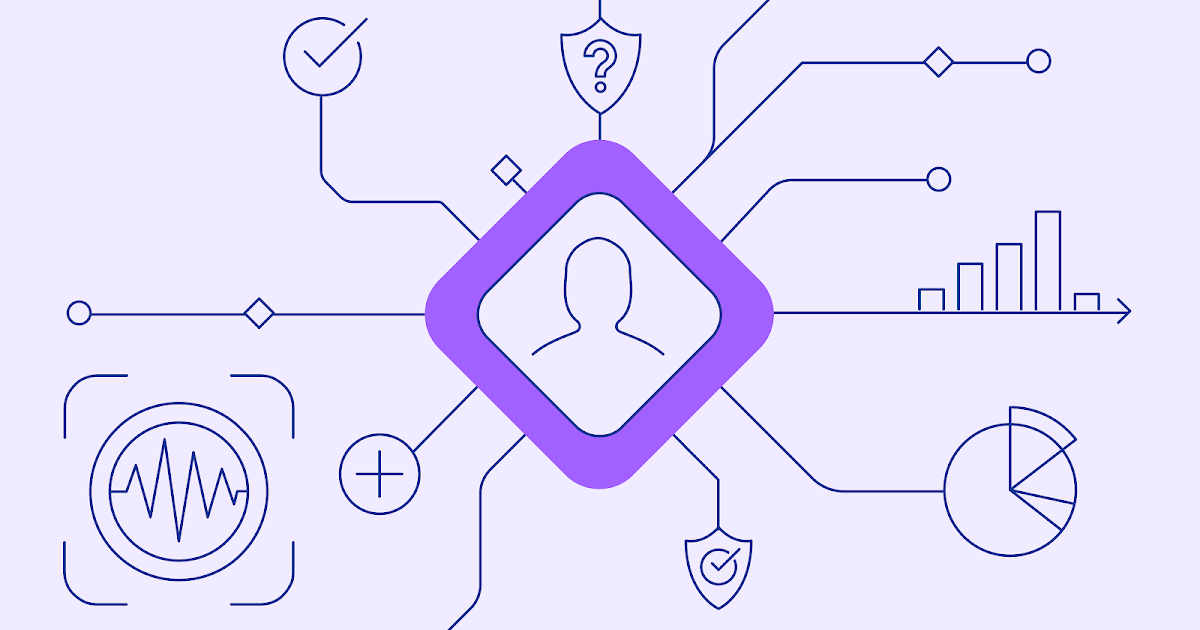
[ad_1]
Building a responsible approach to data collection with the Partnership on AI
At DeepMind, our goal is to make sure everything we do meets the highest standards of safety and ethics, in line with our Operating Principles. One of the most important places this starts with is how we collect our data. In the past 12 months, we’ve collaborated with Partnership on AI (PAI) to carefully consider these challenges, and have co-developed standardised best practices and processes for responsible human data collection.
Human data collection
Over three years ago, we created our Human Behavioural Research Ethics Committee (HuBREC), a governance group modelled on academic institutional review boards (IRBs), such as those found in hospitals and universities, with the aim of protecting the dignity, rights, and welfare of the human participants involved in our studies. This committee oversees behavioural research involving experiments with humans as the subject of study, such as investigating how humans interact with artificial intelligence (AI) systems in a decision-making process.
Alongside projects involving behavioural research, the AI community has increasingly engaged in efforts involving ‘data enrichment’ – tasks carried out by humans to train and validate machine learning models, like data labelling and model evaluation. While behavioural research often relies on voluntary participants who are the subject of study, data enrichment involves people being paid to complete tasks which improve AI models.
These types of tasks are usually conducted on crowdsourcing platforms, often raising ethical considerations related to worker pay, welfare, and equity which can lack the necessary guidance or governance systems to ensure sufficient standards are met. As research labs accelerate the development of increasingly sophisticated models, reliance on data enrichment practices will likely grow and alongside this, the need for stronger guidance.
As part of our Operating Principles, we commit to upholding and contributing to best practices in the fields of AI safety and ethics, including fairness and privacy, to avoid unintended outcomes that create risks of harm.
The best practices
Following PAI’s recent white paper on Responsible Sourcing of Data Enrichment Services, we collaborated to develop our practices and processes for data enrichment. This included the creation of five steps AI practitioners can follow to improve the working conditions for people involved in data enrichment tasks (for more details, please visit PAI’s Data Enrichment Sourcing Guidelines):
- Select an appropriate payment model and ensure all workers are paid above the local living wage.
- Design and run a pilot before launching a data enrichment project.
- Identify appropriate workers for the desired task.
- Provide verified instructions and/or training materials for workers to follow.
- Establish clear and regular communication mechanisms with workers.
Together, we created the necessary policies and resources, gathering multiple rounds of feedback from our internal legal, data, security, ethics, and research teams in the process, before piloting them on a small number of data collection projects and later rolling them out to the wider organisation.
These documents provide more clarity around how best to set up data enrichment tasks at DeepMind, improving our researchers’ confidence in study design and execution. This has not only increased the efficiency of our approval and launch processes, but, importantly, has enhanced the experience of the people involved in data enrichment tasks.
Further information on responsible data enrichment practices and how we’ve embedded them into our existing processes is explained in PAI’s recent case study, Implementing Responsible Data Enrichment Practices at an AI Developer: The Example of DeepMind. PAI also provides helpful resources and supporting materials for AI practitioners and organisations seeking to develop similar processes.
Looking forward
While these best practices underpin our work, we shouldn’t rely on them alone to ensure our projects meet the highest standards of participant or worker welfare and safety in research. Each project at DeepMind is different, which is why we have a dedicated human data review process that allows us to continually engage with research teams to identify and mitigate risks on a case-by-case basis.
This work aims to serve as a resource for other organisations interested in improving their data enrichment sourcing practices, and we hope that this leads to cross-sector conversations which could further develop these guidelines and resources for teams and partners. Through this collaboration we also hope to spark broader discussion about how the AI community can continue to develop norms of responsible data collection and collectively build better industry standards.
Read more about our Operating Principles.
[ad_2]
Source link