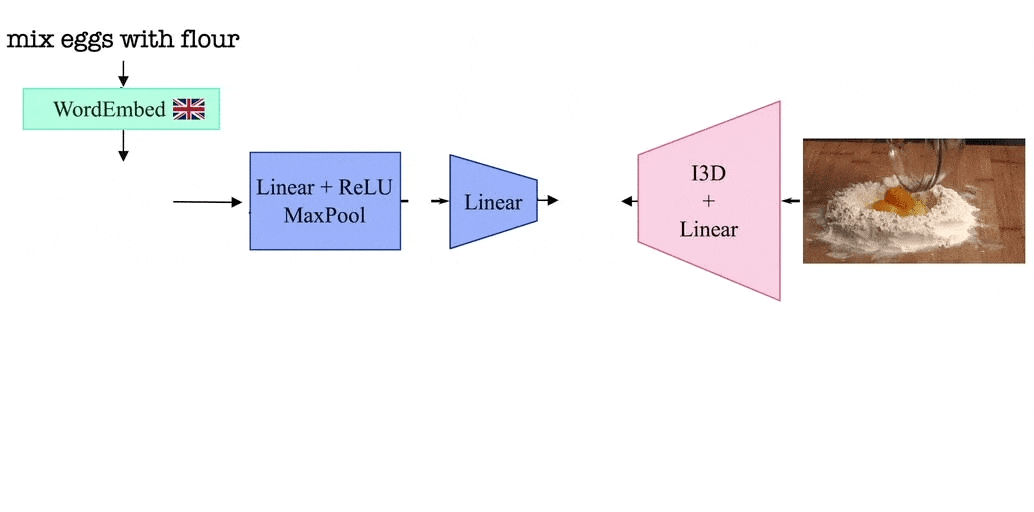
[ad_1]
Translating Words Through Unpaired Narrated Videos
The most common approach for machine translation relies on supervision through paired or parallel corpus where each sentence in the source language is paired with its translation in the target language. This is limiting as we do not have access to such a paired corpus for most languages in the world. Interestingly, bilingual children can learn two languages without being exposed to them at the same time. Instead, they can leverage visual similarity across situations: what they observe while hearing “the dog is eating” on Monday is similar to what they see as they hear “le chien mange” on Friday.
In this work, inspired by bilingual children, we develop a model that learns to translate words from one language to another by tapping into the visual similarity of situations in which words occur. More specifically, our training dataset consists of disjoint sets of videos narrated in different languages. These videos share similar topics (e.g., cooking pasta or changing a tire); for example, the dataset consists of some videos on how to cook pasta narrated in Korean and a different set of videos on the same topic but in English. Note that the videos in different languages are not paired.
Our model leverages the visual similarity of videos by associating videos with their corresponding narrations in a shared embedding space between languages. The model is trained by alternating between videos narrated in one language and those in the second language. Thanks to such a training procedure, and since we share the video representation between both languages, our model learns a joint bilingual-visual space that aligns words in two different languages.
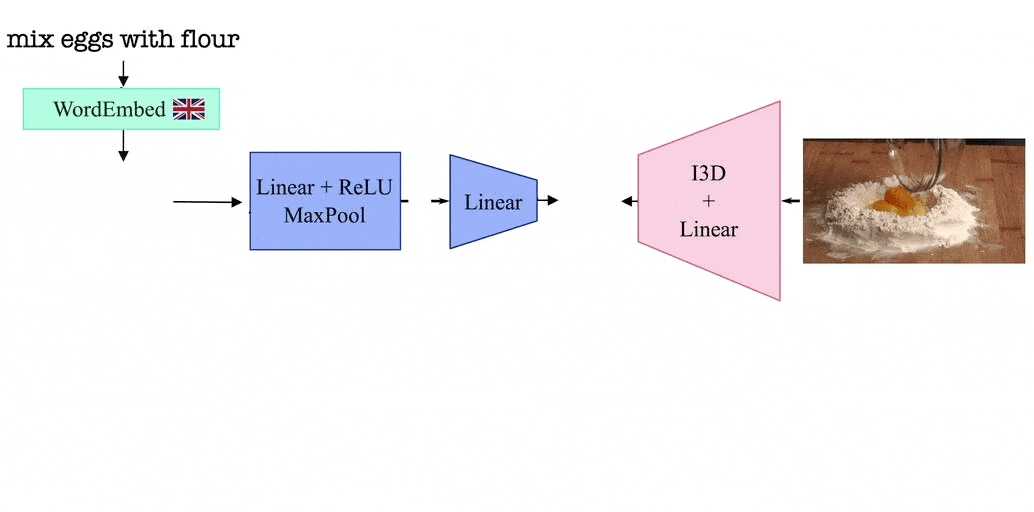
MUVE: improving language only methods with vision
We demonstrate that our method, MUVE (Multilingual Unsupervised Visual Embeddings), can complement existing translation techniques that are trained on unpaired corpus but do not use vision. By doing so, we show that the quality of unsupervised word translation improves, most notably in situations where language-only methods suffer the most, e.g., when: (i) languages are very different (such as English and Korean or English and Japanese), (ii) the initial corpora have different statistics in the two languages, or (iii) a limited amount of training data is available.
Our findings suggest that using visual data such as videos is a promising direction to improve bilingual translation models when we do not have paired data.
[ad_2]