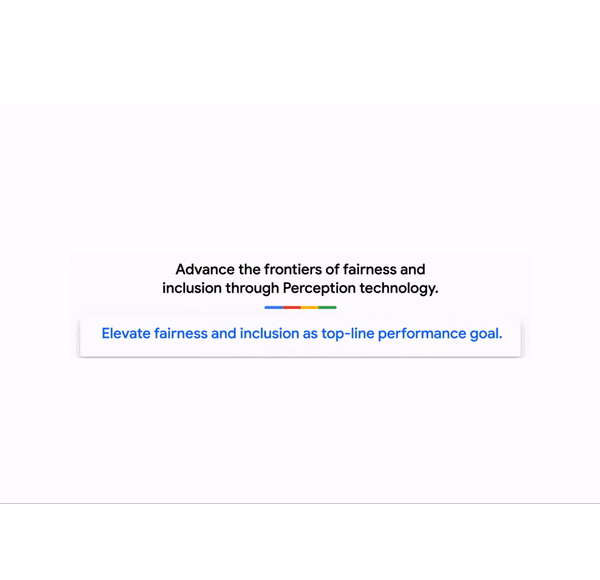
[ad_1]
Google’s Responsible AI research is built on a foundation of collaboration — between teams with diverse backgrounds and expertise, between researchers and product developers, and ultimately with the community at large. The Perception Fairness team drives progress by combining deep subject-matter expertise in both computer vision and machine learning (ML) fairness with direct connections to the researchers building the perception systems that power products across Google and beyond. Together, we are working to intentionally design our systems to be inclusive from the ground up, guided by Google’s AI Principles.
![]() |
Perception Fairness research spans the design, development, and deployment of advanced multimodal models including the latest foundation and generative models powering Google’s products. |
Our team’s mission is to advance the frontiers of fairness and inclusion in multimodal ML systems, especially related to foundation models and generative AI. This encompasses core technology components including classification, localization, captioning, retrieval, visual question answering, text-to-image or text-to-video generation, and generative image and video editing. We believe that fairness and inclusion can and should be top-line performance goals for these applications. Our research is focused on unlocking novel analyses and mitigations that enable us to proactively design for these objectives throughout the development cycle. We answer core questions, such as: How can we use ML to responsibly and faithfully model human perception of demographic, cultural, and social identities in order to promote fairness and inclusion? What kinds of system biases (e.g., underperforming on images of people with certain skin tones) can we measure and how can we use these metrics to design better algorithms? How can we build more inclusive algorithms and systems and react quickly when failures occur?
Measuring representation of people in media
ML systems that can edit, curate or create images or videos can affect anyone exposed to their outputs, shaping or reinforcing the beliefs of viewers around the world. Research to reduce representational harms, such as reinforcing stereotypes or denigrating or erasing groups of people, requires a deep understanding of both the content and the societal context. It hinges on how different observers perceive themselves, their communities, or how others are represented. There’s considerable debate in the field regarding which social categories should be studied with computational tools and how to do so responsibly. Our research focuses on working toward scalable solutions that are informed by sociology and social psychology, are aligned with human perception, embrace the subjective nature of the problem, and enable nuanced measurement and mitigation. One example is our research on differences in human perception and annotation of skin tone in images using the Monk Skin Tone scale.
Our tools are also used to study representation in large-scale content collections. Through our Media Understanding for Social Exploration (MUSE) project, we’ve partnered with academic researchers, nonprofit organizations, and major consumer brands to understand patterns in mainstream media and advertising content. We first published this work in 2017, with a co-authored study analyzing gender equity in Hollywood movies. Since then, we’ve increased the scale and depth of our analyses. In 2019, we released findings based on over 2.7 million YouTube advertisements. In the latest study, we examine representation across intersections of perceived gender presentation, perceived age, and skin tone in over twelve years of popular U.S. television shows. These studies provide insights for content creators and advertisers and further inform our own research.
![]() |
An illustration (not actual data) of computational signals that can be analyzed at scale to reveal representational patterns in media collections. [Video Collection / Getty Images] |
Moving forward, we’re expanding the ML fairness concepts on which we focus and the domains in which they are responsibly applied. Looking beyond photorealistic images of people, we are working to develop tools that model the representation of communities and cultures in illustrations, abstract depictions of humanoid characters, and even images with no people in them at all. Finally, we need to reason about not just who is depicted, but how they are portrayed — what narrative is communicated through the surrounding image content, the accompanying text, and the broader cultural context.
Analyzing bias properties of perceptual systems
Building advanced ML systems is complex, with multiple stakeholders informing various criteria that decide product behavior. Overall quality has historically been defined and measured using summary statistics (like overall accuracy) over a test dataset as a proxy for user experience. But not all users experience products in the same way.
Perception Fairness enables practical measurement of nuanced system behavior beyond summary statistics, and makes these metrics core to the system quality that directly informs product behaviors and launch decisions. This is often much harder than it seems. Distilling complex bias issues (e.g., disparities in performance across intersectional subgroups or instances of stereotype reinforcement) to a small number of metrics without losing important nuance is extremely challenging. Another challenge is balancing the interplay between fairness metrics and other product metrics (e.g., user satisfaction, accuracy, latency), which are often phrased as conflicting despite being compatible. It is common for researchers to describe their work as optimizing an “accuracy-fairness” tradeoff when in reality widespread user satisfaction is aligned with meeting fairness and inclusion objectives.
To these ends, our team focuses on two broad research directions. First, democratizing access to well-understood and widely-applicable fairness analysis tooling, engaging partner organizations in adopting them into product workflows, and informing leadership across the company in interpreting results. This work includes developing broad benchmarks, curating widely-useful high-quality test datasets and tooling centered around techniques such as sliced analysis and counterfactual testing — often building on the core representation signals work described earlier. Second, advancing novel approaches towards fairness analytics — including partnering with product efforts that may result in breakthrough findings or inform launch strategy.
Advancing AI responsibly
Our work does not stop with analyzing model behavior. Rather, we use this as a jumping-off point for identifying algorithmic improvements in collaboration with other researchers and engineers on product teams. Over the past year we’ve launched upgraded components that power Search and Memories features in Google Photos, leading to more consistent performance and drastically improving robustness through added layers that keep mistakes from cascading through the system. We are working on improving ranking algorithms in Google Images to diversify representation. We updated algorithms that may reinforce historical stereotypes, using additional signals responsibly, such that it’s more likely for everyone to see themselves reflected in Search results and find what they’re looking for.
This work naturally carries over to the world of generative AI, where models can create collections of images or videos seeded from image and text prompts and can answer questions about images and videos. We’re excited about the potential of these technologies to deliver new experiences to users and as tools to further our own research. To enable this, we’re collaborating across the research and responsible AI communities to develop guardrails that mitigate failure modes. We’re leveraging our tools for understanding representation to power scalable benchmarks that can be combined with human feedback, and investing in research from pre-training through deployment to steer the models to generate higher quality, more inclusive, and more controllable output. We want these models to inspire people, producing diverse outputs, translating concepts without relying on tropes or stereotypes, and providing consistent behaviors and responses across counterfactual variations of prompts.
Opportunities and ongoing work
Despite over a decade of focused work, the field of perception fairness technologies still seems like a nascent and fast-growing space, rife with opportunities for breakthrough techniques. We continue to see opportunities to contribute technical advances backed by interdisciplinary scholarship. The gap between what we can measure in images versus the underlying aspects of human identity and expression is large — closing this gap will require increasingly complex media analytics solutions. Data metrics that indicate true representation, situated in the appropriate context and heeding a diversity of viewpoints, remains an open challenge for us. Can we reach a point where we can reliably identify depictions of nuanced stereotypes, continually update them to reflect an ever-changing society, and discern situations in which they could be offensive? Algorithmic advances driven by human feedback point a promising path forward.
Recent focus on AI safety and ethics in the context of modern large model development has spurred new ways of thinking about measuring systemic biases. We are exploring multiple avenues to use these models — along with recent developments in concept-based explainability methods, causal inference methods, and cutting-edge UX research — to quantify and minimize undesired biased behaviors. We look forward to tackling the challenges ahead and developing technology that is built for everybody.
Acknowledgements
We would like to thank every member of the Perception Fairness team, and all of our collaborators.
[ad_2]
Source link